Contents
- What is AI Due Diligence?
- AI Due Diligence Trends
- Due Diligence for AI – STATS
- Why AI Due Diligence is Crucial for Businesses?
- Key Components of AI Due Diligence
- A Step-by-Step AI Due Diligence Process
- Best Practices for Streamlining AI Due Diligence
- Ai Due Diligence Checklist
- Challenges in AI Due Diligence
- Conclusion – Future of AI Due Diligence
In today’s rapidly evolving technological landscape, AI due diligence has become a crucial process for businesses, investors, and decision-makers who are engaging with AI-powered technologies. Whether it’s for mergers and acquisitions (M&A), investing in AI startups, or simply integrating AI systems into existing operations, evaluating the potential risks and benefits of AI is essential. AI due diligence ensures that organizations understand the capabilities, limitations, and ethical implications of the technology they are adopting.
As AI becomes more ingrained in daily business operations, understanding its legal, technical, and financial implications can help businesses mitigate risks while optimizing opportunities. In this guide, we’ll walk you through the comprehensive steps and best practices involved in AI due diligence, equipping you with the knowledge to make informed decisions.
What is AI Due Diligence?
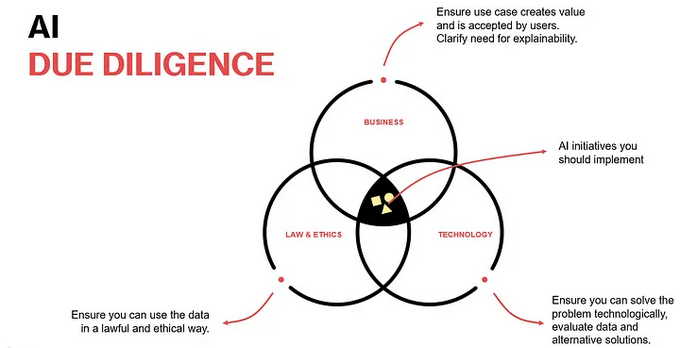
The process of using AI and machine learning tools to enhance the due diligence process, which involves evaluating various aspects before deploying or investing in AI systems. AI Due Diligence assesses risks, benefits, compliance, ethical implications, data privacy concerns, algorithmic biases, and overall impact on stakeholders, providing a comprehensive evaluation framework. This process is particularly valuable for organizations and investors looking to ensure responsible and informed decision-making in the adoption of AI technologies.
The process starts with mapping tasks performed by employees or customers within a chosen business area. The goal is to identify tasks that demand decision making and generate hypothesis on how AI could be used to support that decision making process. Once the long list of hypotheses of potential AI use cases is created, we evaluate them through a three-fold due diligence process:
AI Due Diligence Trends
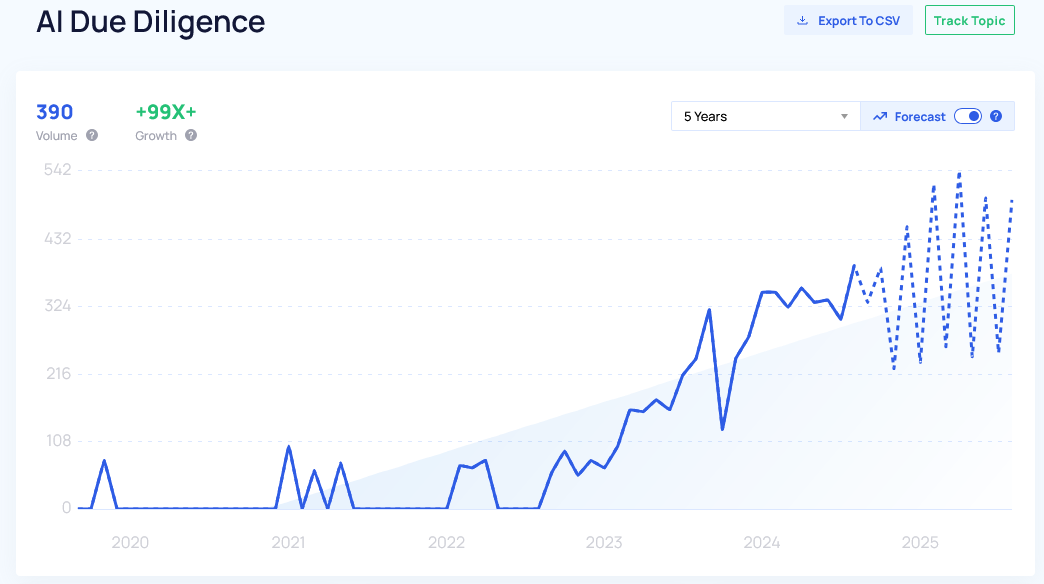
Due Diligence for AI – STATS
- According to a report by PwC, the global market for mergers and acquisitions reached a record high of $3.8 trillion in 2018, with the technology, energy, and healthcare sectors leading the way.
- A study by Ernst & Young found that 56% of mergers and acquisitions fail to meet their original goals due to lack of proper due diligence.
- In a survey by Deloitte, 84% of executives cited due diligence as a critical step in the acquisition process.
- In a survey by KPMG, 27% of companies cited cultural misalignment as the main reason for post-acquisition integration challenges.
- According to a report by Intralinks, 40% of deals fail because of issues uncovered during due diligence.
- In a survey by Grant Thornton, 52% of respondents said they have walked away from a deal because of issues uncovered during due diligence.
- In a survey by Merrill Corporation, 84% of executives said they have encountered at least one issue during due diligence that was not previously disclosed.
- In a study by McKinsey & Company, companies that effectively manage the due diligence process have a 45% higher success rate in meeting their goals for the acquisition.
Why AI Due Diligence is Crucial for Businesses?
As AI technologies increasingly drive innovation across industries, performing AI due diligence becomes essential for mitigating risks and ensuring long-term success. Here’s why:
- Investment Decision Making: Investors and businesses looking to acquire or integrate AI systems must assess their potential value and viability. AI due diligence helps identify the strengths and weaknesses of the technology, ensuring it aligns with organizational goals and delivers a strong return on investment (ROI)
- Risk Mitigation: AI systems bring unique risks, including data security vulnerabilities, algorithmic biases, and compliance issues. By conducting thorough AI due diligence, organizations can uncover hidden risks in areas such as data privacy, intellectual property (IP), and ethical AI practices
- Compliance and Regulation: The legal landscape surrounding AI is complex and continually evolving. Ensuring that AI technologies comply with regulations like GDPR, HIPAA, or industry-specific standards is critical for avoiding legal liabilities and penalties. AI due diligence evaluates compliance with these regulations, helping businesses safeguard themselves from potential violations
AI due diligence not only protects companies from legal and financial pitfalls but also positions them to leverage AI responsibly, ensuring that the technology integrates smoothly with existing processes and delivers optimal results.
Key Components of AI Due Diligence
When conducting AI due diligence, it’s essential to evaluate multiple aspects of the technology to ensure a thorough understanding of its potential and risks. Here are the core components that should be addressed during the process:
Technical Assessment
- Algorithm Evaluation: Understanding the AI model’s underlying algorithms is critical. This includes evaluating their effectiveness, scalability, and ability to adapt to new data. It’s also important to consider how these algorithms were trained and whether there are biases present in the data.
- Data Quality: The performance of any AI system is heavily dependent on the quality of the data it processes. Poor data quality can lead to inaccurate predictions or faulty decisions. During due diligence, you should assess the sources of data, how it is collected, cleaned, and its overall integrity.
Legal and Ethical Review
- Regulatory Compliance: With increasing regulations around AI, ensuring that AI systems comply with data protection laws (such as GDPR) and industry-specific standards is crucial. This includes verifying that the AI system respects privacy rights, handles data securely, and adheres to any ethical AI guidelines.
- Intellectual Property Rights: It’s vital to confirm that the AI technology does not infringe on existing patents or intellectual property. This includes reviewing licenses, patents, and ownership of any proprietary algorithms.
Team & Expertise
Skillset Evaluation: The success of AI largely depends on the team behind it. Evaluating the skills and experience of the developers, data scientists, and engineers responsible for creating and maintaining the AI technology ensures that the system is well-supported and capable of evolving over time).
By thoroughly examining these components, businesses can make well-informed decisions regarding the adoption or investment in AI technologies, ensuring that they choose solutions that are both effective and compliant.
A Step-by-Step AI Due Diligence Process
To ensure a comprehensive evaluation of AI technologies, the AI due diligence process should follow a structured, methodical approach. Below are the essential steps involved:
Define Objectives:
Begin by clearly identifying the purpose of the AI due diligence. This could be for investment, acquisition, or assessing a partnership with an AI-driven organization. Defining the objectives helps to focus the scope and the specific areas of the technology to evaluate.
Assemble an Expert Team:
AI due diligence requires a multidisciplinary team with expertise in AI technologies, data science, legal frameworks, and financial analysis. The team should include technical experts who can evaluate algorithms, legal professionals to assess compliance and IP rights, and financial analysts to measure the economic impact.
Information Collection:
- Documentation Review: Collect technical specifications, data usage policies, and regulatory documents. Obtain access to internal documents, including the AI system’s codebase, data sources, and performance metrics.
- Stakeholder Interviews: Engage key personnel, such as developers, data scientists, and leadership teams, to gather insights into the AI system’s development, goals, and potential challenge.
Technical Assessment:
- Algorithm Evaluation: Review the AI’s underlying algorithms, focusing on their design, accuracy, and ability to handle large-scale data. Ensure that the algorithms are robust, adaptable, and free from significant bias.
- Performance Metrics: Compare the system’s performance metrics (e.g., accuracy, precision, recall) with industry standards and assess whether the system meets the organization’s performance needs.
Risk Evaluation:
- Legal and Ethical Risks: Identify potential compliance risks, including data privacy violations, intellectual property issues, and ethical concerns like AI bias and transparency.
- Technology Risks: Consider risks associated with AI technology failure, system scalability, and data security. Ensure that the system has safeguards in place to mitigate potential threats.
Report and Decision Making:
Compile the findings into a detailed report. Highlight areas of strength, potential risks, and opportunities for improvement. Provide actionable recommendations for moving forward, such as implementing risk mitigation strategies or renegotiating terms based on the due diligence results.
By following these steps, businesses and investors can thoroughly assess the viability of AI technologies and make informed decisions that balance innovation with caution.
Best Practices for Streamlining AI Due Diligence
Conducting thorough AI due diligence can be a time-consuming and complex task. However, following best practices can help streamline the process, making it more efficient and effective:
Utilize AI Tools for Due Diligence:
- AI tools can be used to automate parts of the due diligence process itself, such as document review, data analysis, and risk identification. These tools speed up the process by quickly scanning large datasets and highlighting anomalies or areas that need further investigation.
- For example, Natural Language Processing (NLP) models can automatically review contracts and identify clauses that may pose compliance risks.
Develop a Comprehensive Checklist:
- Having a detailed checklist specific to AI due diligence ensures that no critical aspect is overlooked. This checklist should include categories like technical evaluation, legal compliance, ethical considerations, and financial analysis.
- Prioritize the key components, such as verifying the AI algorithms and checking for legal risks, to focus on the most important areas first.
Ai Due Diligence Checklist
1. Technical Capabilities Assessment
- Evaluate the AI models and algorithms for scalability, adaptability, and performance in different scenarios (e.g., geographic or demographic shifts.
- Review the data quality feeding AI models. Ensure it is representative, properly managed, and capable of evolving over time.
- Assess the technical infrastructure needed to support AI, including cloud architecture and machine learning pipelines.
2. Bias and Fairness
- Investigate if the AI system has been trained on biased data (e.g., gender, race) and what mechanisms exist to correct these biases.
- Confirm whether ongoing bias monitoring systems are in place to identify and mitigate bias.
3. Robustness and Security
- Evaluate the security measures in place to protect AI data from breaches, and check for vulnerabilities to cyberattacks.
- Ensure data encryption and safe handling practices are implemented for both internal and external data sources.
4. Ethics and Compliance
- Ensure that the AI system complies with relevant privacy laws, such as GDPR, CCPA, and other global or local regulations.
- Review policies on data anonymization and user consent for training AI systems.
5. Interpretability and Explainability
- The AI system should provide clear explanations for its decisions, not operate as a “black box.” Confirm that decision-making processes can be interpreted and explained to stakeholders.
- Validate that there are mechanisms to communicate AI outputs effectively to non-technical stakeholders like business managers and regulators.
6. Governance and Responsible AI
- Ensure that the target company has a responsible AI framework in place, including ethical guidelines and accountability standards for AI usage.
- Verify the AI governance system includes constant monitoring for ethical practices and compliance with legal frameworks.
7. Employee Skills and Integration
- Assess whether the employees, beyond data scientists, possess the skills to manage AI systems post-acquisition.
- Create an integration strategy that outlines how AI will be merged into the acquiring company’s operation.
This checklist combines elements from various sources and is meant to provide a well-rounded assessment of AI systems in the due diligence process.
Leverage Data Integration Tools
Integrating various data sources into one platform can streamline the assessment process. By centralizing data from multiple systems—such as financials, technical performance, and compliance records—it becomes easier to analyze and draw insights during the due diligence process.
Continuous Monitoring and Updates:
Due diligence doesn’t end after the initial evaluation. Implement systems for ongoing monitoring of the AI’s performance, compliance with legal standards, and ethical impact. This ensures that the AI system continues to operate effectively and within regulatory bounds.
Phased Implementation:
Start by running a pilot program for evaluating the AI system in smaller, controlled environments. This allows the team to address any initial challenges before a full-scale implementation.
By implementing these best practices, businesses can ensure that their due diligence process is not only comprehensive but also efficient, reducing the time and resources required while ensuring all risks and opportunities are addressed.
Challenges in AI Due Diligence
Data Accessibility: Issues with Accessing Confidential Data for AI Evaluation
One of the primary challenges in AI due diligence is accessing the confidential data necessary for a thorough evaluation. Evaluating AI systems often requires a deep dive into proprietary datasets to understand their quality, diversity, and potential biases.
However, gaining access to this data can be problematic due to privacy concerns, confidentiality agreements, and regulatory restrictions.
According to EY Building Better World, companies must navigate complex legal and ethical considerations when handling sensitive information, which can hinder the due diligence process.
Effective AI due diligence requires robust data-sharing agreements and transparent data governance policies to ensure that all parties have the necessary access while maintaining data security and privacy.
AI’s Legal Limitations: Understanding the Legal Constraints of AI
AI technologies are subject to various legal constraints, including intellectual property rights and compliance with existing regulations. Understanding these limitations is crucial for effective AI due diligence. As highlighted by NeuroSYS and SpotDraft, intellectual property issues can arise when evaluating AI systems, particularly regarding patent rights, copyright, and trade secrets. Additionally, AI systems must comply with local and international regulations, which can vary widely. Companies need to stay informed about the evolving legal landscape to avoid potential legal pitfalls. This requires legal expertise and ongoing monitoring of relevant legislation to ensure that AI implementations adhere to all legal requirements.
Conclusion – Future of AI Due Diligence
The landscape of AI technology is rapidly evolving, and so is the field of AI due diligence. As AI continues to advance, its influence on business decisions and operations will only grow. the future of AI due diligence will likely involve more sophisticated ai software development tools and methodologies to address emerging challenges. Companies will need to adapt their due diligence practices to keep pace with technological advancements and regulatory changes. This includes staying updated on best practices, integrating new evaluation techniques, and fostering a culture of transparency and ethical responsibility.
Implementing thorough AI due diligence practices is essential for making informed decisions in AI-related ventures. As AI technologies become increasingly integral to business operations, it is crucial for organizations to proactively address the challenges associated with evaluating these systems. By adopting comprehensive due diligence strategies, companies can mitigate risks, ensure compliance, and leverage AI technologies effectively. We encourage readers to invest in robust due diligence practices, stay informed about legal and technical developments, and engage with experts to navigate the complexities of AI evaluation.