Enterprise AI vs Generative AI
In the present day and age, Digital Transformation has become the paramount priority in virtually every sector, and this process continues to progress at a rapid pace. Organizations that once relied solely on manual operations are now gradually embracing automated methods. And, all this is possible due to the integration of generative AI .
Since more and more companies start investing in AI, Enterprise AI, and Generative AI have emerged as the two sides of a coin. Now, you might be wondering what exactly are Enterprise AI and Generative AI.
Well, in the short term, we can say that Enterprise AI is a machine learning and artificial intelligence application used for routine business activities, whereas Generative AI utilizes machine learning and artificial intelligence to enable machines to synthesize existing content on the web and generate counterfeit content such as audio, video, text, and images. The benefits of Enterprise AI to society are distinct from those of Generative AI.
Understanding the key differences between these two types of AI is important for businesses looking to leverage AI technologies to enhance their operations. In this article, we will explore the key differences and benefits of enterprise AI and generative AI. So, let’s take a look!
Contents
Enterprise AI vs Generative AI Explained
1. Enterprise AI, also known as business AI, is artificial intelligence that focuses on enhancing business operations, performance, and efficiency. It involves the use of data analysis, machine learning algorithms, and automation tools to solve complex problems and streamline various tasks.
Enterprise AI Benefits
Enterprise AI can assist businesses in enhancing customer support, which can be a powerful tool for driving sales. By leveraging virtual chatbots, monitoring customer behavior, and enhancing customer-business interactions, enterprises can improve their support offerings, and drive sales.
As marketing is a significant contributor to business growth, Enterprise AI can help develop innovative marketing strategies that would be challenging to achieve with traditional techniques. Therefore, businesses can leverage this technology to create a competitive edge in the market and drive growth.
- Enhance Efficiency: Enterprise AI helps to automate repetitive and time-consuming tasks, allowing employees to focus on more critical and creative tasks.
- Improve Decision-Making: By leveraging data analysis, Enterprise AI provides insights and predictions that enable better decision-making and risk management.
- Enhance Customer Experience: With Enterprise AI, businesses can provide personalized and efficient customer service, increasing customer satisfaction.
- Cost Savings: By automating tasks and improving operational efficiency, Enterprise AI helps businesses reduce costs and increase profitability.
- Competitive Advantage: Businesses that adopt Enterprise AI can gain a competitive advantage by optimizing their operations and delivering better products and services to customers.
For instance, AI-powered fraud detection systems can analyze transactions and identify potential fraudulent activity before it occurs.
2. Generative AI, also known as creative AI, is a type of artificial intelligence that focuses on creating new content, such as art, music, or text, that can be used for various purposes. Generative AI uses neural networks, natural language processing, and other techniques to generate new content based on existing patterns and structures. Here are some benefits of Generative AI
Generative AI Benefits
Leveraging Generative AI can enable businesses to automate tasks and save valuable resources such as time and money. Moreover, it can enhance the efficiency of operations, thereby delivering a positive impact on overall business performance.
Notably, one of the key benefits of this technology is the ability to create relevant images aligned with the text in real time, a feature that marketers can leverage to create brand awareness and hype. Beyond efficiency, Generative AI also promises to deliver high-quality output in the form of audio, video, images, and text.
- Improve Creativity: Generative AI can help humans generate new ideas and concepts by providing new perspectives and insights.
- Increase Productivity: By automating the creative process, Generative AI can help artists, musicians, and writers produce more content in less time.
- Personalization: Generative AI can create personalized content tailored to individual preferences and interests, leading to higher engagement and satisfaction.
- Innovation: Generative AI can generate new and innovative solutions to complex problems, leading to breakthroughs in various fields.
- Accessibility: Generative AI can democratize the creative process by making it accessible to a wider audience, regardless of their background or skill level.
For example, a fashion retailer can use Generative AI to create personalized product recommendations for customers, based on their preferences and browsing history.
A graphic designer can use Generative AI to create new design elements, such as textures or patterns, that can be used in various projects.
Both Enterprise AI and Generative AI offer significant benefits for businesses and individuals. By understanding the benefits and limitations of each approach, businesses and individuals can leverage AI to achieve their goals and gain a competitive advantage in today’s rapidly evolving business landscape.
Key Differences Between Enterprise AI & Generative AI
As previously noted, Generative AI and Enterprise AI differ in their approaches to streamlining human tasks. These technologies employ distinct techniques to achieve this goal.
1. Enterprise AI utilizes techniques such as Heuristics, Natural Language Processing (NLP), Machine Learning, Support Vector Machine, Markov Decision Process, and Artificial Neural Networks (ANN).
Heuristics, a technique based on the trial-and-error method, is a prominent technique used in Enterprise AI to solve complex business problems. NLP is a technique utilized by voice assistants to capture and process text, and then convert it into audio. This popular technique is widely used in Microsoft Word to facilitate enterprise activities.
Artificial Neural Networks (ANN) work similarly to natural neural networks and assist enterprises in detecting complex patterns in a given dataset. Machine learning is programmed to learn from previous experiences and execute specific enterprise tasks.
The Markov Decision Process technique is based on the decision-making process and indicates which actions should be taken by the machine in a given situation and at what time.
2. Generative AI employs techniques such as Generative Adversarial Networks (GAN), Transformers, and Variational Auto-Encoders. GANs utilize two neural networks, discriminators and generators, which function in opposition to each other to seek out patterns in the networks.
Transformers in Generative AI are trained to understand images, audio, text, language, and data classification. Wu-Dao, GPT-3, and LAMDA are examples of transformers that measure input data differently based on its importance. The input data undergoes compression into code, which is then decoded by the transformer to produce the actual information. All of this is accomplished via variational auto-encoders.
Enterprise AI & Generative AI Challenges
Generative AI has become prominent and troublesome. With the ability to create convincing models of genuine individuals, Generative AI can be used to perform a variety of crimes. The repercussions of such actions could be fatal, disrupting entire workforces and causing chaos.
Unfortunately, mischievous individuals have already begun to exploit the technology for their own gains, using it to imitate others for disreputable purposes such as blackmail, revenge, or ransom. one of the significant challenges is that people are using it to propagate falsehoods and create fake stories, instead of harnessing its power for constructive purposes.
Enterprise AI’s adoption in the organization is not as easy as one thinks. It comes with many perks, but challenges must also concern the budget. The integration of Enterprise AI is an expensive affair, and small-scale industries are worried about implementing it.
Considerations for selecting Enterprise AI or Generative AI
As businesses across various industries look to leverage Artificial Intelligence (AI) to improve their operations, choosing the right approach can be a challenging task. With Enterprise AI and Generative AI being the two most common approaches, it is essential to understand the considerations for selecting one over the other, as well as the factors that influence the decision-making process.
Here, we will explore both of these topics to help businesses make informed decisions when selecting an AI approach.
- Purpose: The first consideration is the purpose of the AI implementation. Is it to solve specific business problems or create new content? Enterprise AI is best suited for problem-solving, while Generative AI is ideal for Content Strategy.
- Industry: Different industries have unique needs and requirements when it comes to AI. Consider the nature of your business and whether it requires a more problem-solving or content-creation approach.
- Data Availability: The availability and quality of data play a significant role in the selection process. Enterprise AI requires significant amounts of structured data, while Generative AI can work with less structured data.
- Resource Availability: Both approaches require different levels of resources, including financial, technical, and human. Consider whether your business has the resources to implement and maintain the chosen approach.
Factors that influence the decision-making process
Choosing the right AI approach for your business requires careful consideration of various factors. Whether you opt for Enterprise AI or Generative AI, ensure that the approach aligns with your business goals, industry, available resources, and ethical considerations. By doing so, you can leverage the benefits of AI to improve your business operations and stay ahead of the competition.
- Business Goals: The first factor is the business goals that AI aims to achieve. Does the business seek to improve productivity, reduce costs, or create new revenue streams? The choice of AI approach should align with these goals.
- Technical Expertise: Implementing an AI approach requires technical expertise. Consider whether your business has the necessary expertise or whether it needs to outsource to a third party.
- Timeframe: The timeframe for implementing AI can vary depending on the approach selected. Consider the urgency of the business needs and the time available to implement the chosen approach.
- Ethical Considerations: AI can raise ethical considerations, such as data privacy, fairness, and bias. Consider the ethical implications of the AI approach selected and whether they align with the business’s values.
Takeaway
Choosing the right AI approach for your business requires careful consideration of various factors, including your business goals, data availability, budget, and resource availability. Enterprise AI and generative AI are two popular approaches to AI that offer different benefits and challenges.
Enterprise AI is focused on solving problems and improving operational performance, while generative AI is geared toward creating new content. Both approaches can bring significant benefits to your business, including increased efficiency, productivity, and innovation.
It is important to choose the approach that aligns with your business goals, industry, and available resources. By leveraging the power of AI, your business can stay ahead of the competition and unlock new opportunities for growth and success. RedBlink, a leading Generative AI Development Company located in United States, specializes in building generative AI solutions. Our innovative approach and advanced generative AI applications drive growth and fuel innovation.
Are you ready to unlock the true potential of artificial intelligence for your enterprise? Look no further than RedBlink’s AI consulting services. With their deep expertise in Generative AI and related niches, we provide unparalleled guidance and support. From developing customized AI solutions to optimizing existing frameworks, RedBlink empowers businesses to harness the power of cutting-edge technology.
References
- G. Marcus, “Deep Learning: A Critical Appraisal,” arXiv preprint arXiv:1801.00631, 2018.
- S. Ruder, “An Overview of Multi-Task Learning in Deep Neural Networks,” arXiv preprint arXiv:1706.05098, 2017.
- C. Sutton and A. McCallum, “An Introduction to Conditional Random Fields for Relational Learning,” Introduction to Statistical Relational Learning, MIT Press, 2010.
- A. M. Dai and Q. V. Le, “Semi-supervised Sequence Learning,” in Advances in Neural Information Processing Systems (NIPS), pp. 3079-3087, 2015.
- I. Sutskever, O. Vinyals, and Q. V. Le, “Sequence to Sequence Learning with Neural Networks,” in Advances in Neural Information Processing Systems (NIPS), pp. 3104-3112, 2014.
- K. He, X. Zhang, S. Ren, and J. Sun, “Deep Residual Learning for Image Recognition,” in Proceedings of the IEEE Conference on Computer Vision and Pattern Recognition (CVPR), pp. 770-778, 2016.
- H. Zhang, M. Cisse, Y. N. Dauphin, and D. Lopez-Paz, ” Beyond Empirical Risk Minimization,” in International Conference on Learning Representations (ICLR), 2018.
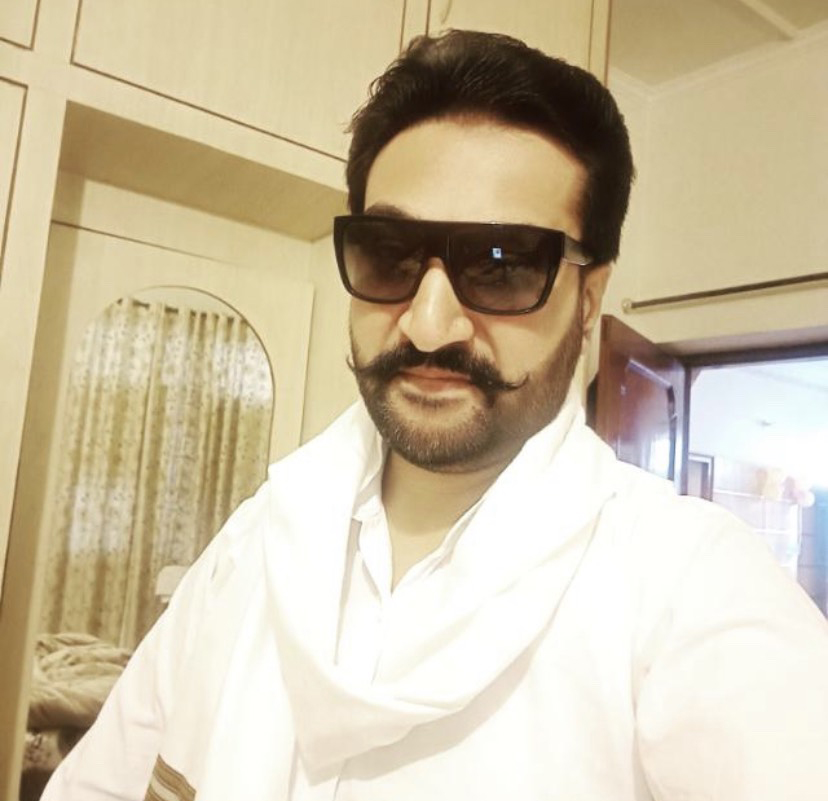
Director of Digital Marketing | NLP Entity SEO Specialist | Data Scientist | Growth Ninja
With more than 15 years of experience, Loveneet Singh is a seasoned digital marketing director, NLP entity SEO specialist, and data scientist. With a passion for all things Google, WordPress, SEO services, web development, and digital marketing, he brings a wealth of knowledge and expertise to every project. Loveneet’s commitment to creating people-first content that aligns with Google’s guidelines ensures that his articles provide a satisfying experience for readers. Stay updated with his insights and strategies to boost your online presence.