Last Updated on September 3, 2024
Have you ever wondered how some businesses consistently stay ahead of market trends, making accurate forecasts that drive their success?
Predicting future trends accurately is a game-changer In the business world. No matter whether you are an SMB or a famous business tycoon, making informed decisions is crucial.
Many of you use traditional forecasting methods, which fall short as business trends are evolving at a rapid pace. It leaves businesses exposed to risks and uncertainties.
This is where predictive modeling works— a sophisticated technique that enhances forecasting accuracy and equips businesses with actionable insights to confidently navigate the future.
According to a study by MarketsandMarkets, the predictive analytics market is expected to grow from $10.5 billion in 2021 to $28.1 billion by 2026, highlighting the increasing reliance on data-driven decision-making.
Here, we explore how predictive modeling can enhance your business forecasting, providing you with the tools and insights to stay ahead.
But before that, let’s understand Traditional Modeling and the reason behind its decreasing popularity.
Contents
- Understanding Traditional Business Forecasting
- What is Predictive Modeling?
- Key Technologies Use in Predictive Modeling
- Key Techniques of Predictive Modelling
- Implementing Predictive Modelling in Business
- Tools and Software
- Future Trends in Predictive Modelling
- Conclusion – Predictive Analytics in Business
Understanding Traditional Business Forecasting
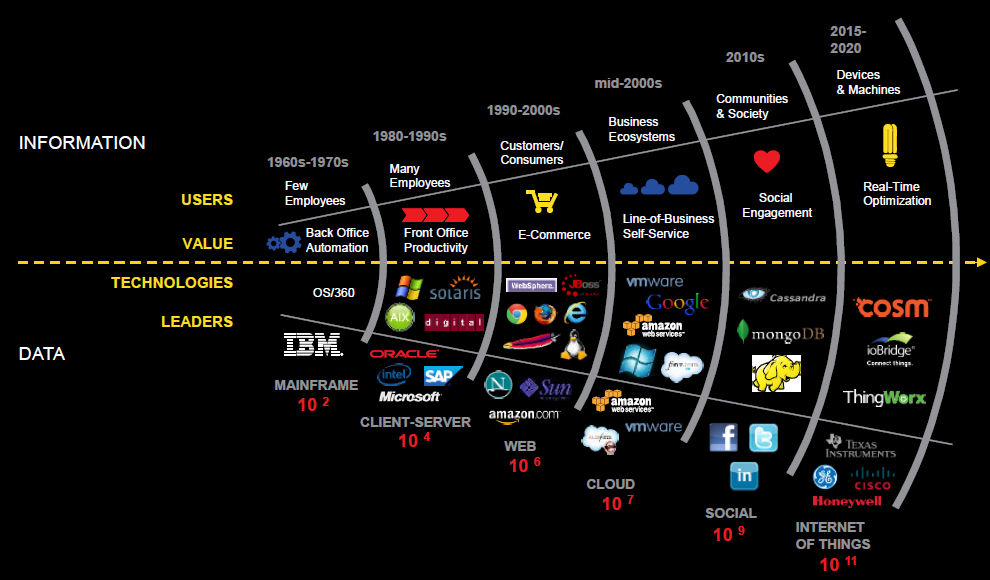
Predictive Analytics in Business – Forecasting Implementation
Traditional business forecasting methods have long been used to predict future business trends based on historical data. While these methods have been foundational in business planning, they have several inherent limitations.
Here’s an overview of the main traditional forecasting methods and their drawbacks:
Traditional Forecasting Method | Definition | Usage | Limitations |
Time Series Analysis | This technique involves analyzing a sequence of data points collected over time to identify patterns or trends. | It is commonly used for forecasting sales, stock prices, and economic indicators. |
|
Linear Regression | A statistical method that models the relationship between a dependent variable and one or more independent variables using a linear equation. | Used to predict outcomes like sales based on factors such as marketing spend or economic conditions. |
|
Moving Averages | A method that smooths out short-term fluctuations and highlights longer-term trends in data. | It is often used in stock market analysis and sales forecasting. |
|
Exponential Smoothing | This method applies decreasing weights to past observations, prioritizing more recent data. | It is effective for short-term trend and seasonal pattern forecasting. |
|
Traditional forecasting methods have their strengths but also come with significant limitations. As businesses face increasingly dynamic and complex environments, these traditional approaches often fall short, making the case for more advanced techniques like predictive modeling.
Now, let’s try to understand what the predictive modeling approach is.
What is Predictive Modeling?
Predictive modeling is a powerful way to forecast future outcomes by analyzing historical data and identifying patterns. It plays a vital role in various business operations, from improving sales forecasts and optimizing inventory to enhancing customer segmentation and risk management.
Recent research highlights the development of digital business forecasting within the ‘Commerce, Culture, and Tourism Plus’ model, utilizing big data and predictive models. This approach integrates various data sources to enhance the accuracy and reliability of business forecasts in these sectors. (source)
Benefits of Predictive Modeling in Businesses:
- Enhanced Decision-Making: Provides data-driven insights, allowing businesses to make more informed decisions.
- Risk Management: Helps identify potential risks and opportunities, facilitating proactive measures.
- Efficiency: Streamlines processes by forecasting demand, optimizing inventory, and managing resources effectively.
Key Technologies Use in Predictive Modeling
-
Integration of AI and Machine Learning
Predictive models became advanced with artificial intelligence (AI) and machine learning (ML) algorithms. These technologies can handle vast amounts of data and identify complex patterns that traditional models might miss, allowing for real-time analysis and decision-making.
This integration significantly boosts the predictive power and model accuracy, making them more sophisticated and reliable.
-
Use of Big Data
The utilization of big data is expanding, providing predictive models with more comprehensive datasets for analysis. By incorporating diverse and extensive data sources, big data enhances the accuracy of predictive models, resulting in more nuanced and reliable forecasts.
Businesses can analyze vast amounts of information to gain deeper insights and make better-informed decisions.
-
Advancements in Natural Language Processing (NLP)
Natural Language Processing (NLP) technologies are improving, allowing predictive models to analyze and interpret human language data more effectively.
This enables businesses to extract insights from unstructured data such as social media posts, customer reviews, and emails, thereby enhancing the predictive power of their models.
As per the given complexity and rapid technological evolution, consulting AI experts can be essential. These experts can help businesses implement advanced predictive models efficiently. They use the latest technologies to boost your return on investment (ROI).
Key Techniques of Predictive Modelling
Predictive modeling involves several vital techniques, each contributing to the accuracy and reliability of the predictions:
-
Regression Analysis
Regression analysis predicts a continuous outcome, like sales or revenue, based on one or more input factors.
How it works: Imagine you’re trying to predict house prices based on the size of the house and its location. Regression analysis finds a mathematical relationship between these factors and the house price.
Use Cases: It’s used for financial forecasting, risk management, and determining pricing strategies.
-
Classification
Classification sorts data into categories. Think of it like sorting emails into “spam” and “not spam.”
How it works: The model looks at features of the data (like email content) and decides which category it fits into.
Use Cases: Customer segmentation, fraud detection, and diagnosing diseases.
-
Clustering
Clustering groups similar data points together without predefined labels.
How it works: If you have customer data, clustering can group customers with similar buying habits together.
Use Cases: Market research, customer profiling, and finding patterns in data.
-
Time Series Analysis
Time series analysis examines data points collected over time to identify trends and patterns.
How it works: If you’re looking at monthly sales data, this method can help you see seasonal patterns and predict future sales.
Use Cases: Stock market predictions, weather forecasting, and inventory management.
These techniques help businesses make better decisions by turning data into actionable insights, making processes more efficient and predictions more accurate.
Implementing Predictive Modelling in Business
Implementing predictive modeling can significantly enhance your business forecasting. Here’s a step-by-step guide to doing it effectively:
Define Objectives:
- The first step is to outline what you want to achieve, such as improving sales forecasts, optimizing inventory, or enhancing customer segmentation. This ensures the predictive modeling aligns with your business goals and provides relevant insights.
Data Collection:
- Once the objective is clear, the next step is to gather data from internal databases, CRM systems, market reports, and external sources. High-quality, relevant data is crucial for accurate predictions. The quality and comprehensiveness of the data collected will significantly impact the accuracy of the predictive model. Ensure that the data is relevant to the problem at hand.
Data Preparation:
- After data collection, data preparation is something that we can’t ignore. Clean and preprocess your data to remove inaccuracies, handle missing values, normalize data, and encode categorical variables. This step is vital for building reliable models.
Choose the Right Model:
- Now, select a model that fits your data and objectives. Regression models are great for predicting continuous outcomes like sales, while classification models sort data into categories, such as identifying fraudulent transactions. Evaluate several models to find the best fit.
Model Training and Testing:
- Once the model is selected, train your model with historical data and test it to see how well it predicts future outcomes. Use metrics like accuracy and precision to evaluate performance. For example, well-trained models can increase predictive accuracy by 30% (source: McKinsey).
Model Deployment:
- Integrate the model into your business processes. Set up systems for real-time data input and make sure decision-makers can access the predictions easily. This step allows immediate application of insights to business operations.
Monitor and Maintain:
- Continuous monitoring of the model’s performance is vital. As new data comes in, periodically retrain the model to maintain its accuracy and relevance. Monitoring helps identify any drifts in the model’s performance and ensures that it adapts to changes in the business environment.
Tools and Software
Choosing the right tools and software can significantly streamline the implementation process. Here are some popular options:
- Python: A versatile programming language with powerful libraries like Pandas, Scikit-Learn, and TensorFlow, making it ideal for data manipulation, machine learning, and deep learning applications.
- R: Known for its strong statistical analysis and visualization capabilities, R is widely used in academic and research settings for predictive modeling.
- SAS: A comprehensive analytics software suite that provides robust data management and advanced predictive modeling features.
- SPSS: An IBM software package used for statistical analysis, which includes predictive analytics capabilities and is user-friendly for those who prefer graphical interfaces over coding.
- RapidMiner: A data science platform that offers an integrated environment for data preparation, machine learning, and model deployment, suitable for users with varying levels of technical expertise.
By leveraging predictive modeling, businesses can transform vast amounts of data into actionable insights, driving strategic decision-making and operational efficiency. Consulting experts in predictive analytics can further boost your return on investment (ROI) by ensuring that models are tailored to your specific needs and industry requirements
Future Trends in Predictive Modelling
Predictive modeling is continuously evolving driven by technological advancements and growing data availability.
Here are some key trends that are shaping the future of predictive modeling:
Increased Adoption Across Industries:
- Trend: More industries are adopting predictive modeling, recognizing its potential to drive business growth and efficiency.
- Impact: Sectors such as healthcare, finance, retail, and manufacturing are leveraging predictive analytics to optimize operations, improve customer experiences, and manage risks.
92%of healthcare leaders surveyed in Singapore reported they had already adopted or are currently in the process of adopting predictive analytics in their healthcare organization, (Source)
Emphasis on Ethical AI and Data Privacy:
- Trend: There is a growing focus on ensuring ethical AI practices and data privacy in predictive modeling.
- Impact: Businesses are implementing guidelines and frameworks to ensure their predictive models are transparent, fair, and compliant with data protection regulations.
Evolution of Predictive Maintenance:
- Trend: Predictive maintenance is becoming more sophisticated, using predictive models to anticipate equipment failures before they occur.
- Impact: This trend is particularly relevant in manufacturing and logistics, where it can significantly reduce downtime and maintenance costs.
Predictive modeling is on the cusp of significant transformation, driven by technological and market trends. Businesses that stay ahead of these trends can harness the power of predictive analytics to drive innovation and maintain a competitive edge.
Conclusion – Predictive Analytics in Business
Enhance your business forecasting with the advanced capabilities of predictive modeling. By integrating AI and machine learning, you can significantly improve the accuracy of your predictions and make data-driven decisions that optimize operations and manage risks more effectively.
RedBlink Technologies offers expert guidance to navigate this transformative journey, ensuring you leverage the full potential of Generative AI and predictive analytics to stay ahead of the competition. Discover how we can elevate your forecasting capabilities. Feel free to contact us today!