ChatGPT 3.5 vs ChatGPT 4
Introduction
GPT-3, developed by OpenAI, has 175 billion parameters and has demonstrated impressive natural language understanding and generation capabilities across a wide range of tasks. As AI technology advances, there is a continuous need for improved language models that can better understand context, reason, and generate more accurate and coherent content. ChatGPT 3.5 and ChatGPT 4 represent hypothetical future iterations with enhanced capabilities, reflecting advances in NLP research and technology.
Feature/Capability | GPT-3 | GPT-4 (hypothetical) |
---|---|---|
Training data | 45 terabytes of text | Significantly more data (possibly 100+ terabytes) |
Model size | 175 billion parameters | Even larger (potentially 1 trillion+ parameters) |
Language understanding | Advanced but with limitations | Improved understanding and context-awareness |
Conversational depth | Limited multi-turn conversations | Better multi-turn conversations |
Task versatility | High, can perform various tasks | Improved versatility in tasks and domains |
Knowledge integration | Up to September 2021 | Updated knowledge with more recent information |
Reasoning capabilities | Limited reasoning and logical thinking | Improved reasoning and logic |
Context sensitivity | Moderate context awareness | Enhanced context sensitivity and adaptation |
Response consistency | Inconsistent responses in long conversations | Better consistency in responses |
Ethical considerations | Some biases, can produce unsafe content | Improved content filtering and ethical awareness |
Energy efficiency | High computational requirements | Potential advancements in efficiency |
Please note that this table is purely speculative and based on potential improvements in natural language processing technologies. The actual features and capabilities of a hypothetical GPT-4 may differ.
Announcing GPT-4, a large multimodal model, with our best-ever results on capabilities and alignment: https://t.co/TwLFssyALF pic.twitter.com/lYWwPjZbSg
— OpenAI (@OpenAI) March 14, 2023
Model Architecture
Both ChatGPT 3.5 and ChatGPT 4 might have a higher parameter count than GPT-3, potentially improving their understanding of complex language patterns and relationships. b. These future models could incorporate novel techniques in deep learning and NLP, improving the efficiency and effectiveness of their underlying architecture.
Training Data and Knowledge Base
ChatGPT 3.5 and ChatGPT 4 would likely be trained on more extensive and diverse datasets, allowing them to better understand various topics and contexts. Incorporating more recent data sources would enable these models to stay up-to-date with current events and recent developments. Regularly updating their knowledge bases would ensure more accurate and relevant responses.
Language Understanding and Reasoning
Both hypothetical models might exhibit improved contextual understanding, enabling them to better grasp the nuances of user input and generate more coherent replies. Enhanced reasoning capabilities could allow these models to perform tasks that require logical thinking and problem-solving more effectively. By better understanding context and user intent, these models could provide more accurate predictions and inferences.
NOTE – Don’t miss out our own AI tools created using chatgpt4 API –
Rewrite AI Content To Human Written
Conversational Depth and Consistency
ChatGPT 3.5 and ChatGPT 4 could be better equipped to handle multi-turn conversations, maintaining context and relevance throughout extended dialogues. Improved consistency across longer conversations would result in more reliable and coherent interactions with users. Enhanced context sensitivity and adaptation could enable these models to better respond to user input in a personalized and contextually appropriate manner.
Task Versatility and Domain Adaptability
Both ChatGPT 3.5 and ChatGPT 4 might be able to perform a broader range of tasks across various domains, demonstrating increased adaptability and versatility. Task-specific outputs might be more precise and accurate, reflecting the models’ improved understanding and reasoning capabilities. These models could be more effective at learning from user input, allowing them to better adapt to individual users’ needs and preferences.
Ethical Considerations and Safety Measures
Addressing biases and ethical concerns is crucial, and these future models could feature improved methods for identifying and mitigating biases in their responses. Enhanced content filtering and moderation techniques might be employed to prevent the generation of harmful or inappropriate content. By incorporating safety measures, these models could reduce the potential for misuse and unintended consequences, ensuring more responsible AI deployments.
Energy Efficiency and Computation Requirements
While both hypothetical models may be larger and more complex, advances in technology and optimization techniques could lead to more energy-efficient and computationally efficient models. b. Improved energy efficiency and model optimization could make these language models more accessible and environmentally friendly.
Real-World Applications and Use Cases
Potential use cases for ChatGPT 3.5 and ChatGPT 4 might include content generation, customer support, virtual assistants, tutoring, translation, and more, with improved capabilities offering better results. Industries and sectors that could benefit from these advancements may encompass healthcare, finance, education, entertainment, marketing, and many others, as improved language models provide more accurate, coherent, and context-sensitive outputs.
Remember, this explanation is speculative, as ChatGPT 3.5 and ChatGPT 4 do not exist as of my knowledge cutoff in September 2021. The points mentioned above are based on potential advancements and improvements in the natural language processing field.
Curiosity is piqued by the astounding capabilities of ChatGPT and the array of advantages it brings. Look no further than this enlightening post called What is ChatGPT – Everything You Need to Know?
How much content can we produce in Chatgpt 4?
If GPT-4 were to be developed with improvements over GPT-3, it would likely be able to generate even larger amounts of high-quality content, surpassing the capabilities of GPT-3.
The quantity and quality of content produced by GPT-4 would depend on several factors, including the model size, training data, architecture, and improvements in natural language processing technologies. Given that GPT-3 is already capable of generating extensive amounts of text, a more advanced GPT-4 could potentially generate even larger volumes of content while maintaining or improving the quality, coherence, and context sensitivity.
However, without specific details on GPT-4’s architecture, it is impossible to provide an accurate estimate of the content generation capabilities of a hypothetical GPT-4.
Conclusion
In summary, ChatGPT 3.5 and ChatGPT 4 represent hypothetical future iterations of language models with potential advancements in areas such as model architecture, training data, language understanding, conversational depth, task versatility, ethical considerations, and energy efficiency.
These advancements could significantly impact natural language processing, pushing the boundaries of AI’s capabilities and applications.
Future developments in AI and language models promise to unlock new possibilities and opportunities, transforming the way we interact with technology and harness its potential.
A generative AI development company, Redblink has a team of ChatGPT developers ready to bring these advancements to life. Contact us today to discuss your project requirements and discover how our experts can help you leverage the power of ChatGPT.
Resources for further reading –
If you are interested in learning more about the current state of AI and natural language processing, including GPT-3, you may find these sources helpful:
- Radford, A., Narasimhan, K., Salimans, T., & Sutskever, I. (2018). Improving Language Understanding by Generative Pre-Training. OpenAI. https://s3-us-west-2.amazonaws.com/openai-assets/research-covers/language-unsupervised/language_understanding_paper.pdf
- Brown, T. B., Mann, B., Ryder, N., Subbiah, M., Kaplan, J., Dhariwal, P., … & Amodei, D. (2020). Language Models are Few-Shot Learners. OpenAI. https://arxiv.org/abs/2005.14165
- OpenAI. (2020). Introducing OpenAI’s GPT-3. https://www.openai.com/blog/openai-api/
- Bender, E. M., Gebru, T., McMillan-Major, A., & Shmitchell, S. (2021). On the Dangers of Stochastic Parrots: Can Language Models Be Too Big? https://faculty.washington.edu/ebender/papers/Stochastic_Parrots.pdf
These sources discuss the development and capabilities of GPT-3 and touch on the challenges and limitations of large-scale language models. They provide insight into the current state of AI research and may help inform your understanding of potential future advancements. However, they do not specifically discuss ChatGPT 3.5 or ChatGPT 4, as these versions are hypothetical and not based on real-world developments.
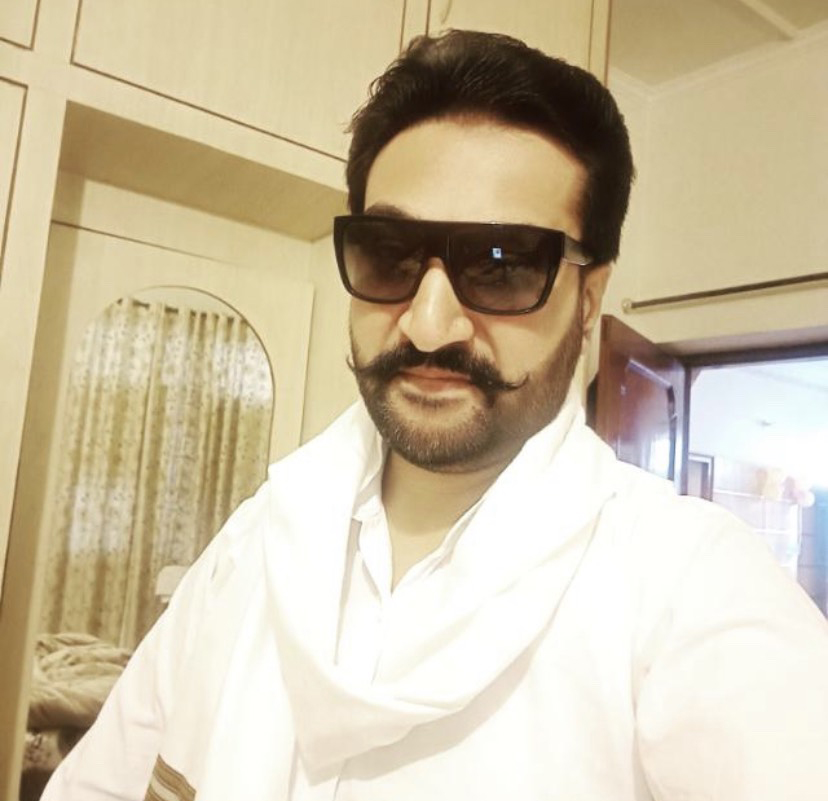
Director of Digital Marketing | NLP Entity SEO Specialist | Data Scientist | Growth Ninja
With more than 15 years of experience, Loveneet Singh is a seasoned digital marketing director, NLP entity SEO specialist, and data scientist. With a passion for all things Google, WordPress, SEO services, web development, and digital marketing, he brings a wealth of knowledge and expertise to every project. Loveneet’s commitment to creating people-first content that aligns with Google’s guidelines ensures that his articles provide a satisfying experience for readers. Stay updated with his insights and strategies to boost your online presence.