How Retrieval-Augmented Generation (RAG) Revolutionizing AI
Artificial intelligence (AI) is more than just a popular term these days; it’s a quickly growing field full of new and exciting technologies that are changing the way we think about AI. One important new technology is called Retrieval-Augmented Generation (RAG).
RAG is set to make big changes in the world of AI. This article will explain RAG in simple terms, showing how it affects Large Language Models (LLMs) like ChatGPT & Google Bard.
We’ll also look at the many ways RAG can be used in different industries, showing that it’s not just a small update, but a major change in AI. By learning about what RAG can do, we can better understand where AI is headed and how it will impact our lives.
Contents
- How Retrieval-Augmented Generation (RAG) Revolutionizing AI
- The Birth of RAG: Facebook’s AI Marvel
- What is RAG?
- Reasons Behind the Origin of RAG AI
- The Role of RAG in Enhancing LLMs
- The Process Behind RAG AI
- Benefits of Retrieval-Augmented Generation
- Real-world Applications of RAG AI
- A Basic RAG Model
- Future of RAG AI
- Get the Best of RAG AI with RedBlink Technologies’ Experienced AI Developers
The Birth of RAG: Facebook’s AI Marvel
Amidst this data deluge, Facebook/Meta has embarked on a journey to create more intelligent NLP models. The result? The Retrieval Augmented Generation (RAG) architecture is poised to redefine the landscape of AI-driven language understanding. This groundbreaking development is now an integral part of the open-source Hugging Face transformer library. At the core of RAG’s innovation is its ability to enhance natural language processing.
What is RAG?
RAG, or Retrieval-Augmented Generation, is a model architecture in the field of natural language processing (NLP) that combines the strengths of two different approaches:
- Retrieval and
- Generation.
This model is designed to improve the quality and relevance of responses in language generation tasks, such as question-answering, chatbot responses, and content creation.
Here’s a breakdown of the two components:
- Retrieval: In this step, the RAG model searches a large database of documents (like Wikipedia or a specialized corpus) to find relevant information based on the input query or prompt. This process is similar to looking up reference materials to gather knowledge about a topic.
- Generation: After retrieving relevant documents, the model uses a generative language model (like GPT-3 or a variant) to compose a coherent and contextually appropriate response. This step involves synthesizing information from the retrieved documents and any additional knowledge the model has learned during its training.
Did you know that retrieval-augmented generation (“RAG”) models combine the powers of pre-trained dense retrieval (DPR) and sequence-to-sequence models?
RAG models retrieve documents, pass them to a seq2seq model, and then marginalize them to generate outputs. The retriever and seq2seq modules are initialized from pre-trained models and fine-tuned jointly, allowing both retrieval and generation to adapt to downstream tasks.
Get in-depth details about RAG AI on Meta’s blog post here–>
Reasons Behind the Origin of RAG AI
The Large Language Model (LLM) sometimes presents false or outdated information and relies on non-authoritative sources, leading to inaccuracies and reduced user trust. Retrieval-augmented generation (RAG) addresses these issues by directing the LLM to authoritative sources, ensuring more accurate and trustworthy responses.
RAG redirects the LLM to retrieve relevant information from authoritative, predetermined knowledge sources. Organizations have greater control over the generated text output, and users gain insights into how the LLM generates the response.
The key advantage of RAG is its ability to pull in up-to-date and specific information from external sources, enhancing the quality and accuracy of its responses. This is particularly useful in scenarios where keeping a language model’s training data current is challenging, such as in rapidly evolving fields or for answering highly specific questions.
The Role of RAG in Enhancing LLMs
RAG enhances LLMs by providing up-to-date information, which ensures timely and relevant responses. Moreover, it reduces misinformation and hallucinations in LLM responses by anchoring them in factual data, which eventually increases the credibility of AI-generated information.
For instance, integration with vector databases like Milvus, a scalable open-source vector database, is essential for RAG’s performance, allowing LLMs to access a verified knowledge base for context in answering questions. This is particularly useful in applications like question-answering systems.
RAG improves domain-specific responses from LLMs by enabling access to relevant databases. Expert-level precision in specialized fields is made possible for LLMs via RAG’s use of domain-specific databases.
Additionally, the construction of an RAG involves critical decisions like data selection, embedding model, and index type. These choices significantly influence the retrieval quality. For example, the text-embedding-ada-002 model demonstrated superior performance in terms of groundedness and answer relevance compared to the MiniLM model, indicating that certain embedding models are more effective for specific types of information like Wikipedia data.
It also enables smarter AI interactions in applications by allowing developers to integrate. As a result, it enhanced user experience and efficiency in applications.
The Process Behind RAG AI
Source: https://www.ml6.eu/blogpost/leveraging-llms-on-your-domain-specific-knowledge-base
1. Advanced Strategies in Data Retrieval:
RAG models are at the cutting edge of databank construction, meticulously gathering data from a wide array of evolving sources. This approach ensures a database that is comprehensive and diverse, offering an all-encompassing perspective.
2. Proficient Interpretation of User Inquiries:
These models excel in unraveling the deeper aims behind user queries. Through an exhaustive dissection of query elements, they achieve an exact understanding, aligning perfectly with the users’ specific needs.
3. Meticulous Data Retrieval Techniques:
Grasping the essence of user objectives, the system leverages cutting-edge algorithms for the precise selection of relevant data. This method prioritizes accuracy, ensuring the extraction of only the most relevant details.
4. Cognitive Enhancement for Increased Precision:
Surpassing standard data retrieval, these models constantly refine their comprehension by merging external, real-world insights. This strategic enhancement significantly heightens the precision and applicability of their responses.
5. Expert Formulation of Responses:
Utilizing a combination of internally sourced data and external insights, these models adeptly craft responses that are both contextually accurate and pertinent. This fusion results in outputs that are not only informative but precisely tailored to the users’ specific inquiries.
Benefits of Retrieval-Augmented Generation
The Retrieval-Augmented Generation (RAG) AI offers a comprehensive range of benefits that significantly enhance both the efficiency and quality of information processing. Here is a complete list of the key advantages:
-
Enhanced Information Extraction:
- Precision and Relevance: RAG’s retrieval models allow for accurate pinpointing of specific details within vast datasets, ensuring high precision and relevance in results.
- Comprehensive Understanding: It can understand and interpret complex queries, extracting nuanced information that traditional models might overlook.
-
Augmented Content Creation:
- Contextual Understanding: RAG grasps and deciphers context, generating coherent content that seamlessly aligns with the surrounding narrative.
- Creativity and Quality: It augments the creative process, producing high-quality content that resonates with the intended audience.
-
Tailored Adaptability:
- Customizable Models: Users can fine-tune Open AI RAG to their specific needs, ensuring optimal performance and relevance for individual business requirements.
- Flexibility: It adapts to various content styles and formats, making it versatile across different industries and content types.
-
Fresh and Contextual Information:
- Up-to-date Data: RAG can tap into information sources that are more current compared to the data used in training traditional Language Models (LLMs).
- Contextual Richness: The knowledge repository of RAG can house fresh and highly contextual data, providing relevant and accurate information.
-
Transparency and Reliability:
- Source Identification: It offers transparency regarding the data sources within its vector database, allowing for quick corrections and updates.
- Data Integrity: This level of transparency ensures high data integrity and reliability, as inaccuracies can be promptly addressed.
-
Improved Decision Making:
- Data-Driven Insights: RAG supports informed decision-making by providing comprehensive and accurate content.
- Analytical Depth: It delves deeper into topics, offering insights and analyses that aid in making well-informed decisions.
-
Scalability and Efficiency:
- Quick Content Generation: Open AI RAG significantly speeds up the content creation process, ideal for scaling up operations and meeting tight deadlines.
- Resource Optimization: It reduces the need for extensive human intervention, optimizing resource allocation and saving time.
-
Continuous Learning and Improvement:
- Adaptive Learning: RAG continually improves its output based on interactions and feedback, ensuring that the content remains relevant and up-to-date.
- Evolving with Trends: It adapts to changing trends and preferences, keeping the content fresh and engaging.
-
Enhanced User Interaction:
- Interactive Experience: RAG can provide more interactive and engaging user experiences, responding accurately to user queries and needs.
- Personalized Responses: It can tailor responses and content to the individual user, enhancing personalization and relevance.
Real-world Applications of RAG AI
- Conversational AI: RAG adapts to various conversational scenarios, offering accurate and contextually relevant responses, essential in sectors like healthcare, finance, and legal services.
- Healthcare: RAG AI in medicine or healthcare, is quickly becoming a major factor in healthcare, building generative AI solutions across all segments, from providers to public health agencies.
- Content Creation: RAG improves efficiency, accuracy, and adaptability in content creation. It simplifies the research process, as exemplified by financial analysts and students using several RAG-powered tools for quick, comprehensive responses.
- Customer Support: HubSpot research shows that 93% of customers remain loyal to companies providing excellent customer support. RAG enhances customer support by integrating real-time, enterprise-specific information, leading to improved accuracy, personalization, streamlined data access, and faster response times. It’s notably effective in sectors like healthcare and technology, ensuring data relevance and timeliness.
- Fact-Checking: In the age of information, fact-checking and precision are of utmost importance. RAG serves as a guardian of truth, capable of verifying the accuracy of factual statements and claims. This ensures that the information presented to users not only remains reliable but also upholds a commitment to truth.
- Programming: For programmers and developers, RAG becomes an invaluable tool. It assists in generating code snippets, documentation, and examples tailored precisely to the context of the programming task at hand. This support streamlines the coding process, enhancing efficiency and reducing errors. Furthermore, several RAG AI-based no-code platforms make the creation of websites and apps a lot easier even for a layman.
- Scientific Research: In the domain of scientific research and discovery, RAG stands as a source of inspiration. It can swiftly and accurately analyze extensive volumes of scientific literature and data, uncovering hidden patterns, emerging trends, and even potential breakthroughs that might otherwise remain concealed.
- Legal Research and Analysis: RAG can assist legal professionals by quickly analyzing extensive legal documents and cases, and identifying precedents, relevant laws, and legal strategies.
- Language Translation and Localization: RAG can be employed to improve machine translation systems by providing contextually accurate translations and ensuring that the translated content is culturally sensitive and contextually relevant.
- Virtual Assistants and Personalized Recommendations: RAG-powered virtual assistants can provide personalized recommendations for products, services, entertainment, and more, based on an individual’s preferences and behaviors.
- Marketing and Advertising: RAG AI in marketing can be used to create more targeted and engaging marketing content, optimize ad campaigns, and improve customer engagement strategies.
- Human Resources and Talent Acquisition: RAG can streamline the recruitment process by screening resumes, conducting initial candidate interviews, and providing insights into the suitability of candidates for specific roles.
- Travel and Tourism: RAG can offer personalized travel recommendations, suggest itineraries, and provide historical and cultural information about travel destinations.
- Data Analysis and Business Intelligence: RAG can assist in analyzing large datasets, identifying patterns, and generating actionable insights for businesses across various industries.
A Basic RAG Model
1. Initial Setup:
- Preparing the environment with necessary libraries.
- Gathering relevant datasets.
- Selecting a pre-trained language model.
- Setting up a retrieval database.
2. Model Training:
- Fine-tuning with specific datasets.
- Integrating retrieval mechanisms.
- Evaluating performance.
3. Advanced Configurations:
- Optimizing hyperparameters.
- Implementing cross-validation.
- Enhancing scalability.
- Incorporating multimodal capabilities.
Future of RAG AI
RAG AI, by integrating with the generative AI capabilities, offers a powerful synergy. This combination allows for more informed and context-rich AI responses, significantly enhancing user experiences. As we look to the future, we can expect RAG AI to become even more sophisticated, with its ability to pull from expansive and dynamic knowledge bases in real-time, offering answers and insights that are both accurate and highly relevant to the user’s context.
In decision-making scenarios, RAG AI’s impact is profound. It promises to streamline complex processes by offering data-driven insights and recommendations, reducing the time and effort needed to sift through large volumes of information. This capability is particularly vital in fields like healthcare, finance, and law, where precision and accuracy are paramount.
Furthermore, RAG AI is expected to be pivotal in shaping a more efficient and intelligent future. Its continuous learning and adaptation capabilities mean that the systems powered by RAG AI will become more robust and effective over time. This ongoing evolution will see AI becoming an even more integral part of our daily lives, from simplifying routine tasks to aiding in complex problem-solving scenarios.
In essence, the future of RAG AI is not just about advanced technology; it’s about creating a more interconnected, intelligent, and accessible world. As this technology matures, we stand on the brink of a new era where AI is not just a tool, but a partner in our quest for knowledge and efficiency.
Get the Best of RAG AI with RedBlink Technologies’ Experienced AI Developers
At RedBlink Technologies, as a generative AI development company our cadre of seasoned AI developers possesses the expertise needed to harness the capabilities of RAG for your Gen AI applications. We recognize that each organization comes with its unique data requisites, and we specialize in constructing tailor-made solutions that eradicate the risks associated with misinformation and outdated data.
Our Methodology:
- Collaborative Engagement: We engage closely with your organization to gain a comprehensive understanding of your specific needs and data sources.
- Customized Strategies: Our team of experts formulates bespoke prompts and retrieval processes, ensuring optimal accessibility to information.
- Continuous Enhancement: We maintain an ongoing process of refinement and updates to your GenAI application, ensuring it remains current and reliable.
In a landscape where the precision of AI-driven solutions is of utmost importance, partnering with us guarantees that you can leverage this groundbreaking technology to its fullest potential, ensuring that your Gen AI applications consistently stay at the forefront of innovation.
RAG represents not merely a buzzword but a new era in AI interaction. Reach out to us today to explore how AI can catalyze a revolution within your organization’s initiatives.
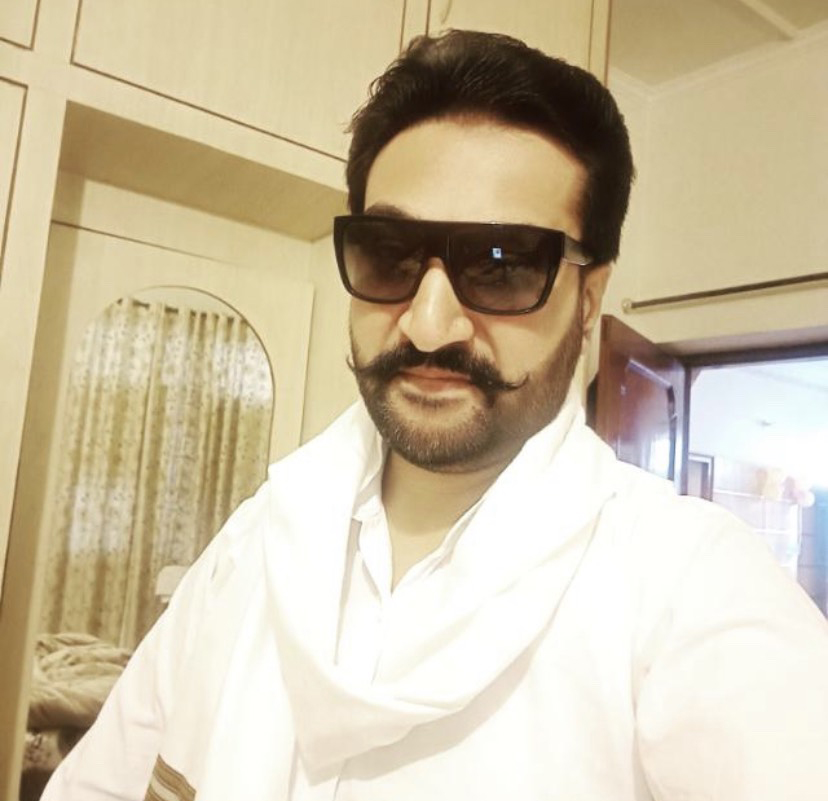
Director of Digital Marketing | NLP Entity SEO Specialist | Data Scientist | Growth Ninja
With more than 15 years of experience, Loveneet Singh is a seasoned digital marketing director, NLP entity SEO specialist, and data scientist. With a passion for all things Google, WordPress, SEO services, web development, and digital marketing, he brings a wealth of knowledge and expertise to every project. Loveneet’s commitment to creating people-first content that aligns with Google’s guidelines ensures that his articles provide a satisfying experience for readers. Stay updated with his insights and strategies to boost your online presence.